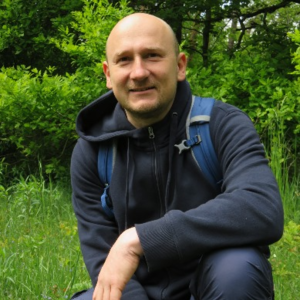
Dr. rer. nat. Michael Schirrmann
Department: Agromechatronics
Program areas
Projects
- AgriNose – Elektronische Nasen für das Monitoring landwirtschaftlicher Flächen basierend auf miniaturisierten Arrays von Affinitätsensoren. TP3: Einsatz von UAV-basierten, fernerkundlich-optischen Methoden zur Detektion von Krankheiten in Winterrogge…
- BioMonitor4Cap – Advanced biodiversity monitoring for results-based and effective agricultural policy and transformation Nicht nachhaltige landwirtschaftliche Praktiken sind die Hauptfaktoren, die die Lebensraum- und Artenvielfalt in den Agrarlandsch…
- BETTER-WEEDS – Wissensbasierte Standortanalyse für ein umweltgerechtes Unkrautmanagement im integrierten Pflanzenbau Derzeit erfolgt die Unkrautkontrolle in konventionellen Ackerbausystemen überwiegend durch angepasste Herbizidstrategien. Vor dem Hin…
- weed-AI-seek – Entwicklung eines intelligenten UAV-gestützten Unkrautmonitoringsystems für den selektiven und teilflächenspezifischen Herbizideinsatz Die Zielsetzung des Projekts weed-AI-seek ist es, ein intelligentes echtzeitfähiges Monitoring- und…
- PHLIP – Entwicklung eines smarten 4D-lnsektenmonitorings für einen integrierten Pflanzenschutz im Erwerbsobstbau (PHLIP) Ziel des Verbundvorhabens ist es ein modularisiertes mobiles System zu entwickeln, welches ein erstes raum-zeitlich hochaufgelöst…
- SustInAfrica – Sustainable intensification of food production through resilient farming systems in West & North Africa Große Teile landwirtschaftlicher Nutzflächen in West- und Nordafrika sind stark degradiert und leiden unter Wasserknappheit, gering…
- Deutsch-Chinesischer Workshop – Internationaler Workshop zum Einsatz smarter Sensoren auf kleinen Betrieben in China und Deutschland - zur Steigerung der Nachhaltigkeit und Produktivität der bäuerlichen Landwirtschaft Vom 30. Juni bis zum 5. Juli org…
- CORONA-pro – Entwicklung einer flugrobotergestützten Expertenplattform für einen präzisen Pflanzenschutz im Erwerbsobstbau Effizienter Pflanzenschutz erfordert eine an die vorhandene Blattfläche angepasste Dosierung der auszubringenden Pflanzenschutz…
- FungiDetect – Sensorgestützte online Detektion von Krankheiten im Getreide. Teilprojekt 1. Ziel des Projekts ist die Entwicklung eines sensorgestützten praxistauglichen Verfahrens zur frühen Erkennung von Gelbrostnestern in Winterweizen. Optische Fah…
Publications
- Aghsaei, H.; Darvishi, A.; Yousefi, M.; Schirrmann, M.; Fohrer, N.; Wagner, P. (2025): Quantifying the Impact of Land Use/Land Cover Change Scenarios on Water-Related Ecosystem Services. Water Resources Management. : p. 1-25. Online: https://doi.org/10.1007/s11269-025-04244-0
- Hobart, M.; Pflanz, M.; Tsoulias, N.; Weltzien, C.; Kopetzky, M.; Schirrmann, M. (2025): Fruit Detection and Yield Mass Estimation from a UAV Based RGB Dense Cloud for an Apple Orchard. Drones. (1): p. 60. Online: https://doi.org/10.3390/drones9010060
- Shamshiri, R.; Sturm, B.; Weltzien, C.; Fulton, J.; Khosla, R.; Schirrmann, M.; Raut, S.; Hanike Basavegowda, D.; Yamin, M.; Hameed, I. (2024): Digitalization of agriculture for sustainable crop production: a use-case review. Frontiers in Environmental Science. : p. 1-32. Online: https://doi.org/10.3389/fenvs.2024.1375193
- Hobart, M.; Schirrmann, M.; Abubakari, A.; Badu-Marfo, G.; Kraatz, S.; Zare, M. (2024): Drought Monitoring and Prediction in Agriculture: Employing Earth Observation Data, Climate Scenarios and Data Driven Methods; a Case Study: Mango Orchard in Tamale, Ghana. Remote Sensing. (11): p. 1942. Online: https://www.mdpi.com/2072-4292/16/11/1942
- Darvishi, A.; Yousefi, M.; Schirrmann, M.; Ewert, F. (2024): Exploring biodiversity patterns at the landscape scale by linking landscape energy and land use/land cover heterogeneity. Science of the Total Environment. : p. 170163. Online: https://doi.org/10.1016/j.scitotenv.2024.170163
- Alirezazadeh, P.; Schirrmann, M.; Stolzenburg, F. (2023): A comparative analysis of deep learning methods for weed classification of high-resolution UAV images. Journal of Plant Diseases and Protection. : p. 227-236. Online: https://doi.org/10.1007/s41348-023-00814-9
- Salamut, C.; Kohnert, I.; Landwehr, N.; Pflanz, M.; Schirrmann, M.; Zare, M. (2023): Deep Learning Object Detection for Image Analysis of Cherry Fruit Fly (Rhagoletis cerasi L.) on Yellow Sticky Traps. Gesunde Pflanzen. (1): p. 37-48. Online: https://doi.org/10.1007/s10343-022-00794-0
- Alirezazadeh, P.; Schirrmann, M.; Stolzenburg, F. (2023): Improving Deep Learning-based Plant Disease Classification with Attention Mechanism. Gesunde Pflanzen. (1): p. 49-59. Online: https://doi.org/10.1007/s10343-022-00796-y
- Tang, Z.; Wang, M.; Schirrmann, M.; Dammer, K.; Li, X.; Brueggeman, R.; Sankaran, S.; Carter, A.; Pumphrey, M.; Hu, Y.; Chen, X.; Zhang, Z. (2023): Affordable High Throughput Field Detection of Wheat Stripe Rust Using Deep Learning with Semi-Automated Image Labeling. Computers and Electronics in Agriculture. (April): p. 107709. Online: https://doi.org/10.1016/j.compag.2023.107709
- Li, M.; Shamshiri, R.; Weltzien, C.; Schirrmann, M. (2022): Crop Monitoring Using Sentinel-2 and UAV Multispectral Imagery: A Comparison Case Study in Northeastern Germany. Remote Sensing. (17): p. 4426. Online: https://doi.org/10.3390/rs14174426