Artificial Intelligence, Data Science and Digital Twins
Digital tools for the bioeconomyInformation-driven processes are increasingly finding their way into agri-food systems and hence into the bioeconomy.
Satellites, mobile and stationary sensors and sensor systems already supply huge amounts of data that are currently mostly used for specific applications and linear value chains.
Understanding and harnessing highly complex interactions
A sustainable and circular bioeconomy is based on highly complex interactions and utilisation paths of a multitude of processes, products and residues.
Our target is to understand the complexity of these highly interconnected and interacting bioeconomic production systems and to make them usable with the help of knowledge-based, dynamic and adaptable process control. Artificial intelligence (AI), Data Science (DS) and Digital Twins (DT) are elementary tools that support us in evaluating the huge amounts of data generated, in developing solutions and in making them applicable in practice.
Artificial intelligence …
AI technologies can be used to analyse large quantities of biological data and data from the technical and natural environment from which patterns and complex correlations can be identified in order to derive independent solutions for a decision or further work steps. AI applications can already be found in practice today, for example in the form of autonomous feeding and milking robots as well as tractors and harvesters that are able to perform their work processes autonomously and can adapt to changing conditions.
AI has the potential to significantly increase the efficiency, sustainability and innovative power of bioeconomic processes. Moreover, AI is also expected to generate innovative solutions to global challenges such as climate change, food security and resource scarcity.
… transparent and comprehensible?
Machine learning methods support a more precise prediction of biological processes and the intelligent automation of procedures. But how verifiable are the results that AI calculates from known source data and does the training data represent the real situation?
At ATB, one research focus is on explainable artificial intelligence and the development of methods that enable a holistic understanding of the behaviour of an AI model. The use of so-called "Bayesian neural networks", for example, provides information about the uncertainties of the decisions made by the AI system and then subsequently presents them in a way that is comprehensible to humans. If, for example, the relevant areas in an image that have contributed significantly to the decision of the AI system are marked in the image analysis, individual decisions of the system can be understood and possible misbehaviour can be reduced.
Digital Twins
Digital Twins are virtual replicas of entire systems with a bidirectional connection to the physical control system. The ability to simulate the real system and receive feedback from this real system makes Digital Twins a powerful tool for tackling highly complex (control) systems and strategies. So far, Digital Twins have mostly been applied to industrial systems with clearly defined start and end points and precisely described causal dependencies of physical or chemical effects and closed process control loops.
Sustainable circular bioeconomy systems the pose some new challenges for the development of Digital Twins. This is because a multitude of different living organisms are also involved in the production and conversion of biomass at the interface of biology and technology. These systems are also bound to very different distinct site conditions and are characterised by a high degree of spatial-temporal heterogeneity, variability and individuality. For sustainable circular bioeconomy systems, it is necessary to manage natural, often stochastic processes as well as to cover a wide range of feedback loops from real-time to long-term reactions. Legal implications should also be considered.
These particularities of sustainable circular bioeconomy systems place high demands on the complex tasks of sensing, data acquisition, data analysis, knowledge generation, modelling, simulation, decision making and automated feedback in the development of Digital Twins. To develop functional and reliable Digital Twins for applications in sustainable circular bioeconomy systems, a wide range of interdisciplinary expertise is required: from data science to agricultural engineering, from sensor technology to microbiological and physiological processes, from embedded programming to process simulation, from hardware design to life cycle assessment.
Some examples from our research
Crop production
At ATB, we research on the use of AI for example to recognise specific weeds in the field (projects weed-AI-seek, Better-Weeds), to identify diseases and pests quickly and at an early stage in order to be able to combat them in a targeted manner (PräzisionsPS) or to be able to automatically recognise hidden obstacles in grassland and cereal crops with AI-based signal processing (MIKIM).
Here, the focus is on methodological issues of intelligent image analysis and the development of smart sensors and sensor networks for the digital recording of environmental conditions as well as the condition of soil, plant and product. These digital methods contribute to the further development of sensor-based process control and automation - a prerequisite for the control of complex systems within a circular bioeconomy.
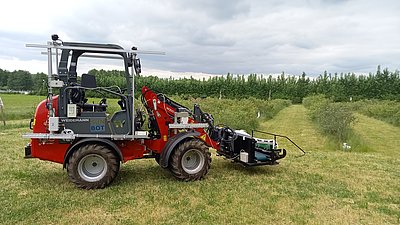
The interaction of AI and robotics is also becoming an increasingly important aspect of our research (e.g. JaetRobi, MIKIM).
A robot recently developed at the ATB (SunBot project, Youtube-Video) will enable farmers in the future to carry out care measures in shrubbery plantations in an automated and energy-autonomous way.
Livestock farming
In livestock farming, AI can help to analyse the health data of farm animals, for example, in order to control housing conditions in the sense of more animal welfare, to better detect stress conditions and infectious diseases, and to better understand the mechanisms of transmission and mitigate the spread of antimicrobial resistance into the environment.
We use data science methods for monitoring, surveillance, simulation and automation in real-time models and bidirectional information flow (DT) to control, for example, the barn climate in naturally ventilated dairy barns and to develop digital solutions for improved decision-making in animal husbandry. (e.g. projects KAMI, DigiMuh, InnoRind, HorseWatch, DaVaSus)
In the RES4LIVE project, we are using Digital Twins to develop an energy-efficient hybrid system for climate control in naturally ventilated dairy barns to effectively reduce heat stress. In order to predict the animals' reaction to heat stress, we use known mechanistic models and combine them with sensor data-based algorithms (informed machine learning). For this purpose, we integrate physiological, behavioural and performance-related reactions of the cows to heat stress and identify sensitive heat stress indicators with their threshold values via non-linear relationships. This information is used to control the air flow and cooling of the supply air. Simulation technology helps us to generate better data on heat transport in the area where animals are present and thus optimise ventilation systems both a priori and with regard to their control and regulation.
... some more examples
Based on existing sensor- and model-based approaches, we develop fully functional Digital Twins for adaptive and highly automated process control.
For example, we are developing a digital twin for an intelligent drying system based on a hybrid control model. This is intended to improve drying processes in terms of product quality and energy efficiency.
For the area of biomass production, we are developing AI-based models based on data analysis and modelling of LiDAR and image data with the aim of creating a Digital Twin to help precisely determine the biomass potential of agroforestry systems (TreeDigitalTwins project).
Joint Lab Artificial Intelligence and Data Science (JL-KI.DS)
In April 2023, the ATB and the University of Osnabrück (UOS) launched the Joint Lab Artificial Intelligence and Data Science (JL-KI.DS).
The aim of the joint research graduate school is to combine the expertise of the UOS in the fields of artificial intelligence, data analysis, law and systems science with the expertise of the ATB in the fields of agricultural, natural, environmental and engineering sciences, among others, in order to develop technologies for sustainable circular bioeconomy systems.
In the JL-KI.DS, young talents receive intensive technical support from experienced scientists with expertise in the fields of agricultural engineering, technology assessment, bioeconomy and data science.
Selected research projects on the topic
-
Using AI technologies and unlocking their potential opens up new opportunities to sustainably intensify milk production and use resources efficiently and competitively. AI tools and data-based applications enable a bette…
-
The aim of the project is the individualised and breeding-based prevention of heat stress in dairy cows with the help of the development of an early warning system and a health monitoring system that alerts the farmer in…
-
The aim of the collaborative project is to develop a modularized mobile system, which will enable an initial spatio-temporal high-resolution population monitoring (4D) of insect populations in orchards, in order to imple…
-
The objective of the weed-AI-seek project is to develop an intelligent real-time monitoring and mapping system for the detection of weed distribution in cereal stands. For this purpose, high-resolution aerial image data …
-
The aim of the joint project TreeDigitalTwins is to develop innovative AI methods for object recognition and automatic derivation of object parameters in discrete 4D point clouds and to test these methods using the examp…
Search for more projects on the topic ...
Ausgewählte Publikationen
- Alirezazadeh, P.; Schirrmann, M.; Stolzenburg, F. (2023): Improving Deep Learning-based Plant Disease Classification with Attention Mechanism. Gesunde Pflanzen. (1): p. 49-59. Online: https://doi.org/10.1007/s10343-022-00796-y
- Salamut, C.; Kohnert, I.; Landwehr, N.; Pflanz, M.; Schirrmann, M.; Zare, M. (2023): Deep Learning Object Detection for Image Analysis of Cherry Fruit Fly (Rhagoletis cerasi L.) on Yellow Sticky Traps. Gesunde Pflanzen. (1): p. 37-48. Online: https://doi.org/10.1007/s10343-022-00794-0
- Dammer, K. (2023): Methoden zur Erkennung des Kartoffelkäfers (Leptinotarsa decemlineata (Say))mit Multispektral- und Farbbildkamera-Sensoren. Gesunde Pflanzen. (1): p. 13-23. Online: https://doi.org/10.1007/s10343-022-00765-5
- Hempel, S.; Adolphs, J.; Landwehr, N.; Janke, D.; Amon, T. (2020): How the selection of training data and modeling approach affects the estimation of ammonia emissions from a naturally ventilated dairy barn - Classical statistics versus machine learning. Sustainability. (3): p. 1030. Online: https://doi.org/10.3390/su12031030
- Jedermann, R.; Singh, K.; Lang, W.; Mahajan, P. (2023): Digital twin concepts for linking live sensor data with real-time models. Journal of Sensors and Sensor Systems. (1): p. 111-121. Online: https://doi.org/10.5194/jsss-12-111-2023
Search for more publications on the topic ...